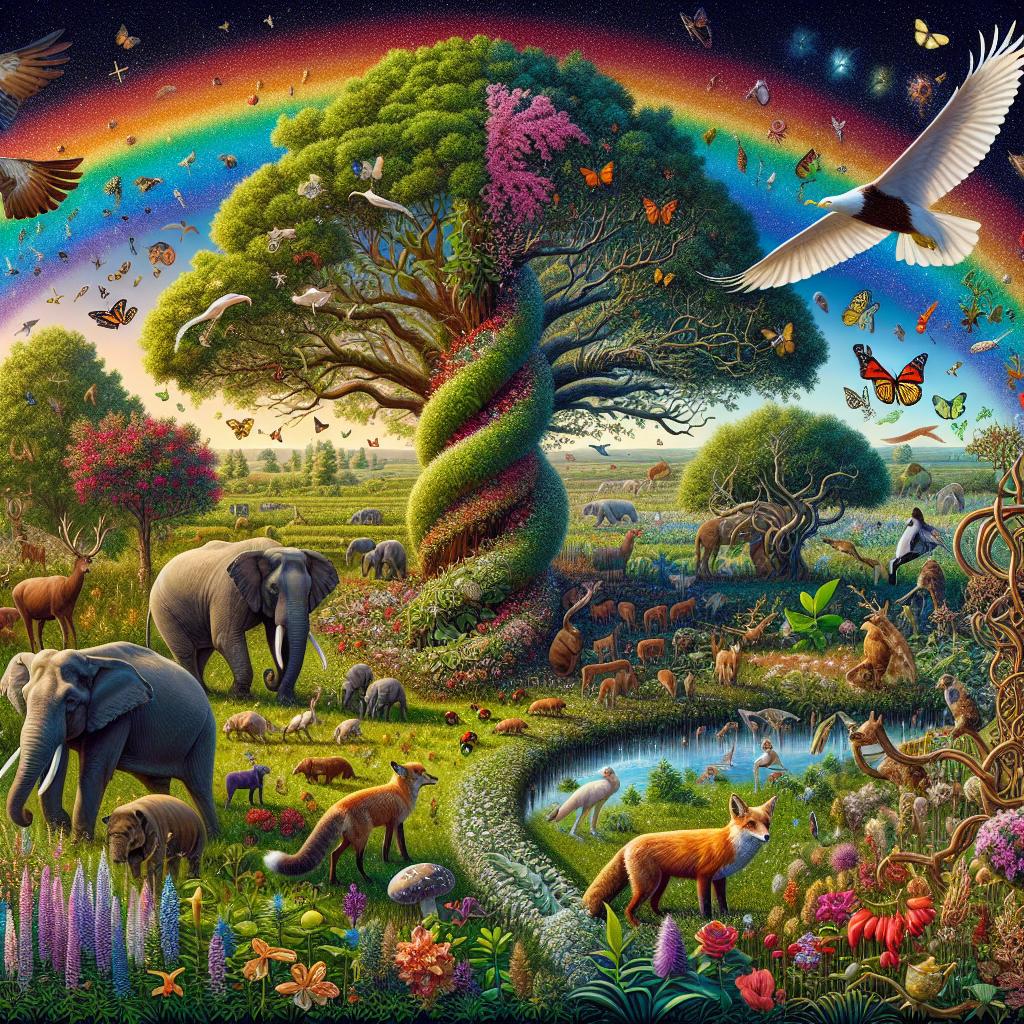
Divine Insights: Unveiling the Truth Behind Genetic Algorithms and Creationism
Published: 28 July 2024
Genetic Algorithms: Do They Show that Evolution Works?
Genetic algorithms (GAs) are computer programs that attempt to simulate biological evolution. GAs have been used in engineering to generate innovative solutions, such as designing electronic circuits with specific functions. Some proponents of evolution argue that GAs demonstrate how biological evolution can create the necessary information for organisms to evolve from simplicity to complexity.
However, it is important to note that GAs do not truly mimic or simulate biological evolution because they have several inherent limitations:
1. Limited Trait Variation
GAs can only select for quantitative traits, where variations can be gradually selected for based on their proximity to the desired objective. This is in contrast to many biological traits, which are qualitative and either work or do not work. Therefore, GAs cannot account for how organisms transition from non-functionality to functionality.
2. Limited Trait Selection
GAs can only select for a limited number of traits, while many complex organisms require hundreds of traits to be viable and survive. In nature, selection operates on all traits that impact an organism's survival.
3. Artificial Preservation of Best Traits
In GAs, the best traits from previous generations are artificially protected from mutations or recombination if no better traits are produced in the next iteration. This ensures that the GA will generate the desired outcome, whereas in real evolution, there is no guarantee that some organisms will always remain viable regardless of mutations.
4. Perfect Selection and High Reproduction Rates
GAs often use perfect selection, where only the best individuals survive to reproduce in each generation. In reality, selection coefficients are much lower, and it would take numerous generations for information-adding mutations to spread through a population. Additionally, GAs commonly produce hundreds or thousands of offspring per generation, whereas most organisms have limited reproductive capabilities.
5. Ignoring Generation Time
GAs operate in microseconds, while real organisms have significantly longer generation times. Multicellular organisms, in particular, have much longer generation times compared to bacteria.
6. Artificially High Mutation Rates
GAs use artificially high mutation rates, which can be sustained because the "genome" is small and the best organism is protected from mutations. In real organisms, such high mutation rates would result in non-viable offspring. Living organisms have sophisticated mechanisms to minimize copying errors during cell division.
7. Artificially Small and Specialized Genomes
GAs typically employ small genomes that only serve a single purpose. However, real organisms have genomes with millions of base pairs and hundreds of proteins coded. Even if a GA were to generate a small amount of information, it would pale in comparison to the complexity of real biological systems.
8. Ignoring Genome-Wide Effects
In real organisms, mutations occur throughout the genome, affecting multiple traits simultaneously. GAs ignore this complexity by only mutating target sequences while protecting the rest of the program. However, an organism's reproduction machinery is not shielded from mutations.
9. Ignoring Irreducible Complexity
Many biological traits require multiple components to function together for the trait to exist. GAs do not account for this irreducible complexity observed in protein synthesis, DNA replication, blood clotting, and other essential biological processes.
10. Ignoring Complex Genetic Interactions
GAs overlook polygeny (traits determined by multiple genes) and pleiotropy (one gene affecting multiple traits). They also ignore recessive genes, which require multiple generations to establish a new trait in a population. These factors contribute to Haldane's Dilemma, which questions the viability of evolution due to limited time for slow-reproducing organisms.
It is crucial to understand that GAs cannot adequately simulate biological evolution due to their inherent limitations. The information space required for even a minimal organism is immense, and naturalistic iterative processes could not account for its development. Furthermore, claims of GAs generating new information overlook the challenges faced by real-world organisms and their limited capacity to generate significant amounts of specified complexity.
Why This Matters
Understanding the limitations of genetic algorithms helps us critically evaluate claims that they demonstrate the plausibility of evolutionary mechanisms. By recognizing the disparities between GAs and real-world evolution, we can engage in more informed discussions about the origins and complexities of life.
Think About It
Consider how the inherent limitations of genetic algorithms impact their ability to accurately simulate biological evolution. Reflect on the vast amount of information present in living organisms and consider how such complexity might challenge evolutionary explanations.